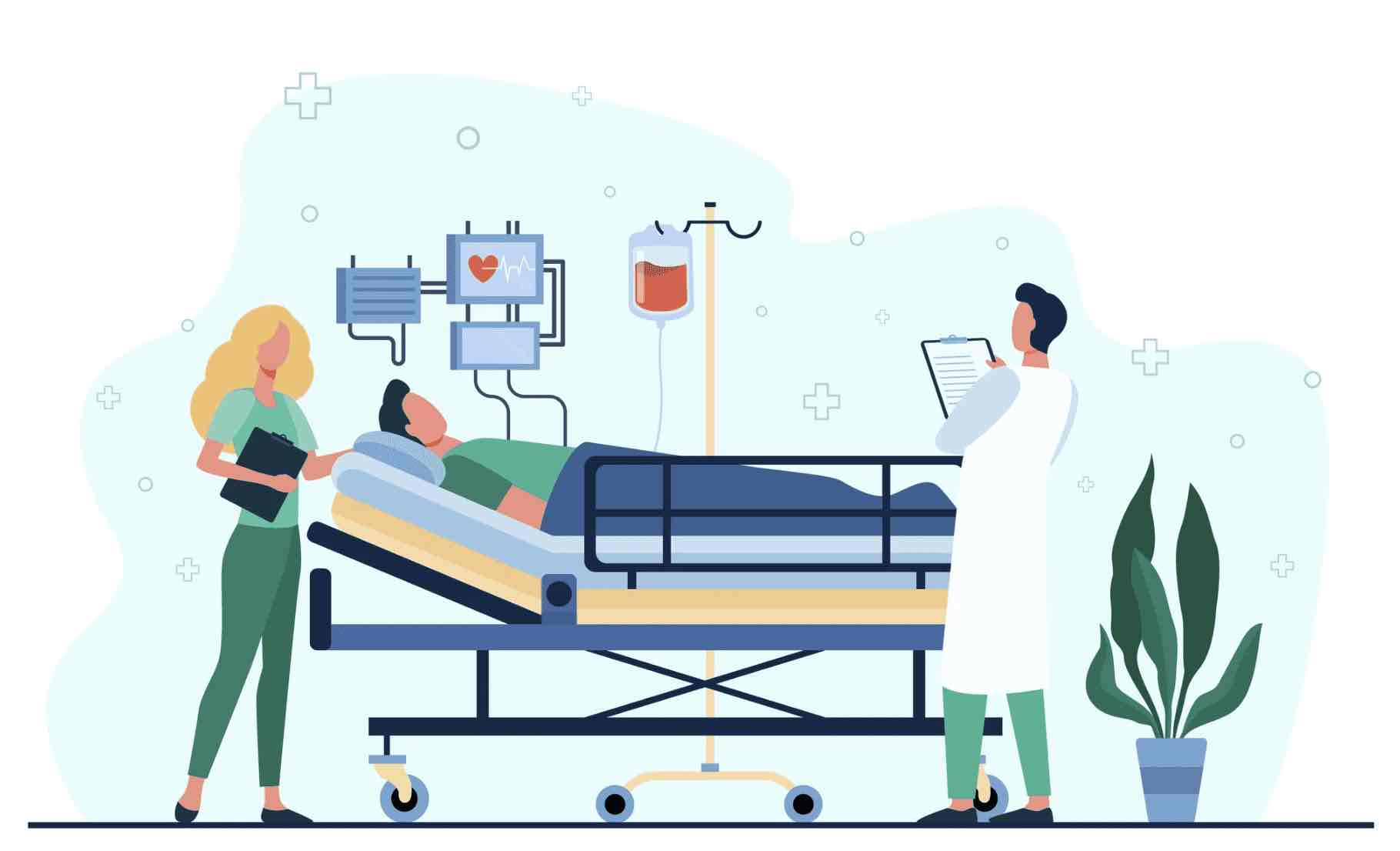
Introduction
Artificial intelligence and ambulatory surgical centers have the potential to revolutionize healthcare & improve patient care exponentially. Across the United States, ambulatory surgery centers (ASC) have offered various surgeries in an outpatient setting since 1970. Their efficiency, affordability, and success rate have seen these health care organizations grow, and they occupy a significant market share today.
Now, artificial intelligence (AI) technology looks set to improve the performance of ambulatory surgery centers (ASC) even further. Here is what patients and surgeons can look forward to.
Artificial Intelligence (AI) has begun to revolutionize the landscape of ambulatory surgical centers (ASCs), bringing forth significant improvements in efficiency, safety, and patient outcomes. By integrating AI-driven tools and systems, ASCs can enhance preoperative assessment, streamline scheduling, and optimize resource allocation. Advanced machine learning algorithms enable real-time analysis of patient data, identifying potential complications, and recommending tailored treatment options.
AI-powered surgical robots assist surgeons in performing precise and minimally invasive procedures, reducing patient recovery time and overall healthcare costs. As AI technology continues to advance, its integration within ASCs will undoubtedly yield increasingly sophisticated solutions, bolstering the quality of care and reshaping the future of outpatient surgery.
Also Read: Robotic Surgery
What are Ambulatory Surgery Centers (ASC)?
Ambulatory surgery centers are health care facilities with fully equipped operating rooms to perform surgeries without admitting patients to a hospital setting. They provide high-quality care more cost-effectively and conveniently. The majority of ambulatory surgery centers (ASC) offer surgeries in several disciplines.
Patients attending an ambulatory surgery centers (ASC) have already visited their primary care practices and been evaluated and diagnosed by a primary health care provider. They are only attending the facility for their surgery and post-anesthesia care and recovery under the supervision of qualified medical personnel.
Key Terms in Ambulatory Surgery Centers
Here are four key terms that are associated with ambulatory surgery centers (ASC).
- Surgicenter or outpatient surgery center are alternative terms for ambulatory surgical centers.
- Ambulatory denotes a condition that can be treated with hospital admission or a procedure that is suitable for selection for outpatient surgery
- Dedicated operating rooms (minimum one) are a prerequisite for accreditation as an ambulatory surgery center (ASC).
- Elective surgeries are those that will benefit a patient but are not urgently required.
Accreditation and Ownership
The Joint Commission on Accreditation of Healthcare Organizations (JCAHO) is responsible for accrediting ambulatory surgery centers. At the moment, the commission recognizes facilities in nine different categories:
- Multi-specialty surgery centers
- Endoscopy centers
- Plastic surgery centers
- Ophthalmology practices
- Laser eye surgery centers
- Centers for oral and maxillofacial surgery
- Orthopedic surgery centers
- Cosmetic and facial surgery centers
- Podiatry clinics
Ambulatory Surgery Centers During pandemic and Future Pandemics
The coronavirus pandemic put temporary restrictions on elective surgeries. As a result, numerous ambulatory surgery centers had to close. As they are reopening, the demand for surgeries carried out in an outpatient setting will likely grow because patients recognize the facilities as safe and efficient. Another reason for their growing popularity is the fact that ambulatory surgery centers (ASC) have been approved by the Centers for Medicare & Medicaid Services (CMS), making it easier to secure reimbursement.
While it is difficult to predict if future pandemics would shut ambulatory surgery centers down once again, managers expect an increased caseload for the coming years. Not only are ambulatory surgery centers becoming more popular with patients, but they are also dealing with clearing the previous pandemic’s case backlog. Block time utilization will be critical to achieving that.
Also Read: When Was the First Robotic Surgery?
How is AI revolutionizing Ambulatory Surgery
Across different industries and subject matters, artificial intelligence and machine learning approaches have proven their unparalleled capacity to handle large amounts of data. In short, technology beats humans at analyzing more data more accurately and in less time than humans can. Observational studies also show that AI and ML excel at recognizing patterns and potential areas for efficiency.
Recent research by scientists at the University of California San Diego considered specifically how these capabilities could be used to optimize time and space use in ambulatory surgery centers (ASC) without compromising patient care. Using retrospective data, a group of researchers set out to develop machine learning models that would predict how often two outcomes coincided: (1) surgery finished by the end of the allocated operating room block time, and (2) the patient was discharged from the post-anesthesia care unit by the end of the relevant nursing shift.
If those two criteria coincided in a so-called composite outcome, it could free up previously unused surgical block time which could then be filled. As a result, ambulatory surgery centers (ASC) could clear the backlogs faster, and more patients would benefit. The researchers hypothesized that the model performance of ensemble learning models would beat that of logistic regressions and their decision functions. Ensemble learning models combine the algorithms and predictive variables of several separate models to make more accurate predictions than individual models can.
To test their theory, they used several different approaches and classifiers. These included regression, random forest classifiers using decision trees, balanced random forest, balanced bagging models, neural network classifiers, and support vector classifiers.
Their analysis also covered the following features: type of surgery, surgeon, service line, and American Society of Anesthesiologists score but not anesthesiologist identification. Other independent features included demographic variables like age, sex, patient weight, and scheduled case duration. The impact of these features was ranked on a feature importance graph. The model performance was evaluated using the synthetic minority oversampling technique (SMOTE).
During their analysis, the scientists looked at more than 13,000 procedures, comparing how the allocated procedure duration days before surgery compared to the actual surgery time and actual room time needed. Actual time was measured in preoperative time, combining the actual case duration and the stay in the post-anesthetic care unit. These retrospective studies showed that the balanced bagging classifier performed best at predicting correct outcomes. Random forest, balanced bagging, and balanced random forest classifiers all delivered more accurate predictions with the SMOTE technique as compared to without.