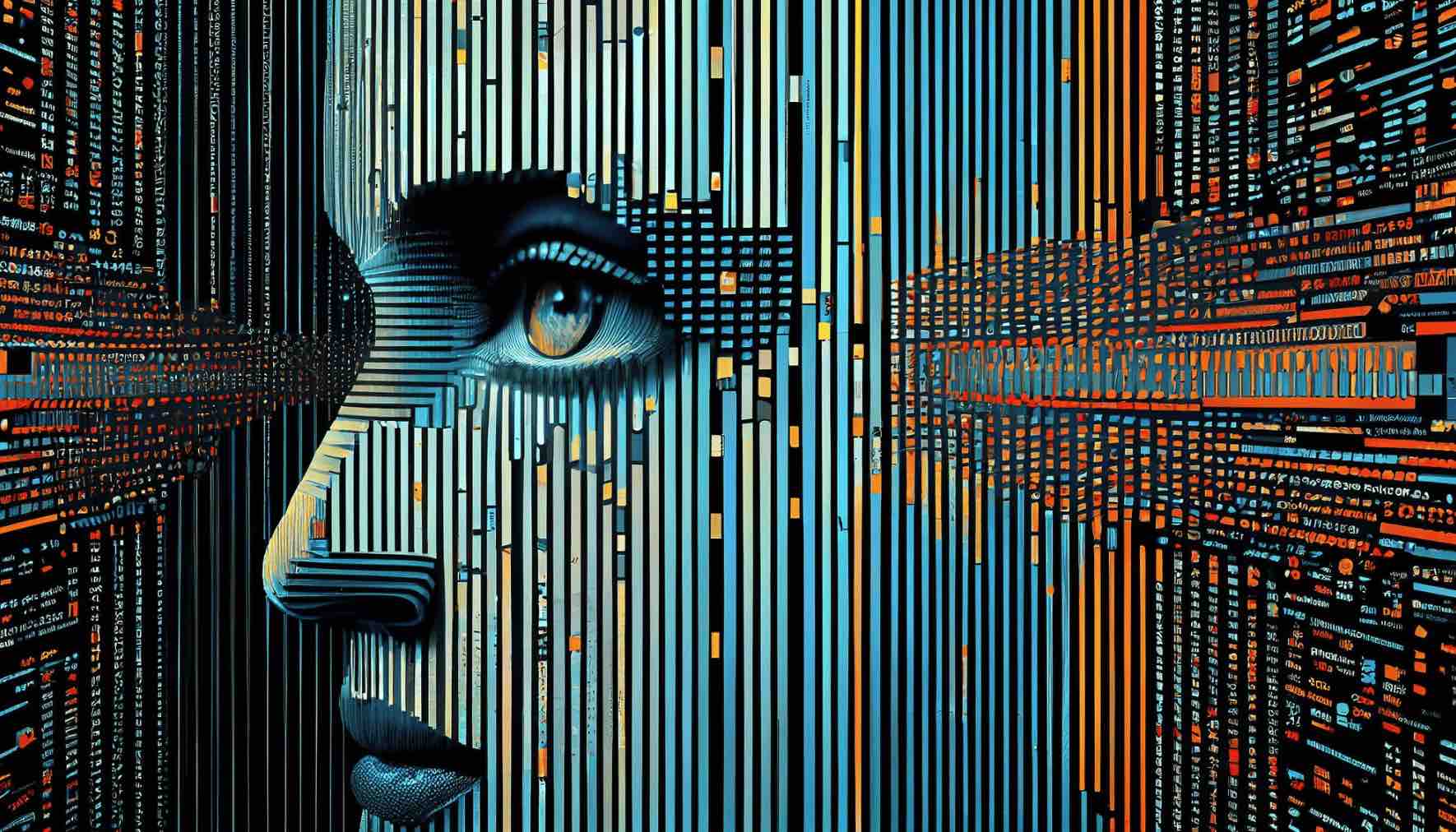
Introduction to Generative AI
Generative Artificial Intelligence (AI) represents a branch of machine learning focused on the production of new data that mirrors the structure and intricacies of the input data. Unlike discriminative models, which classify or differentiate between existing categories, generative models synthesize novel examples of data.
This form of AI is an innovation in the area of machine learning, extending beyond the traditional paradigms that focus solely on data analysis or pattern recognition. While earlier AI applications were designed to interpret and make decisions based on existing data, generative AI leverages algorithms to create new content.
Also Read: How and When Will AI Replace My Job?
Table Of Contents
The Evolution of Generative AI?
Generative AI has undergone an astounding metamorphosis since its nascent stages. The initial iterations of generative AI models were fairly elementary, generally suited for creating basic sequences or elemental patterns. These were not well-suited for the wide range of applications that modern generative AI is employed in. As machine learning models evolved and grew more complex, so did the capabilities and potential applications of generative models.
The transformative moment came with the incorporation of deep learning techniques, which provided the architectural backbone for more intricate and capable generative systems. One particularly impactful development was the inception of Generative Adversarial Networks (GANs). These networks not only advanced the field significantly but also became the cornerstone for many generative AI tools we see today.
Language models, for example, have been fine-tuned to generate text that is virtually indistinguishable from that created by humans. Likewise, foundation models in AI have emerged as a versatile base for developing various types of content, from text to images and beyond.
In our current digital age, generative AI has found resonance across an impressive spectrum of industries. Healthcare professionals use generative AI tools to create detailed medical images, while content creators leverage them for anything from composing music to generating unique visuals in product design.
The ability to produce original content of high quality has made generative AI indispensable in today’s world. The types of content it can create are diverse, catering to a range of applications that was once unimaginable. Whether it’s drafting journalistic reports, formulating artistic concepts, or aiding in scientific research, generative AI has become a ubiquitous and invaluable tool across various disciplines.
Also Read: The Rise of Intelligent Machines: Exploring the Boundless Potential of AI
How Generative AI Works?
Generative models operate through complex algorithms that emulate aspects of human intelligence to learn the intricate features of input data. This learning process is iterative, involving multiple cycles of training and evaluation. During this phase, the model employs various machine learning techniques, most notably leveraging neural networks.
These neural networks are bio-inspired architectures that seek to replicate some aspects of how the human brain processes information. In recent years, we’ve seen the emergence of Generative Pre-trained Transformers (GPT) as a powerful example of such architectures, designed for a multitude of tasks beyond just data generation.
The core of training generative models lies in data—often vast amounts of it. These datasets can either be labeled, which is especially helpful for supervised learning, or unlabeled for unsupervised learning scenarios. The model’s algorithms sift through this information, fine-tuning their internal mathematical parameters.
The goal is to generate output, such as realistic images or text, that faithfully mirrors the nuances and complexity of the original dataset. Pre-trained transformers are particularly useful here, as they have already undergone initial training on large datasets and can, therefore, generate high-quality outputs more efficiently.
Upon completing the training phase, generative models are subjected to rigorous validation and testing processes. The objective is to gauge the model’s effectiveness in generating output that is both structurally coherent and complex, much like the data it was trained on. This is the stage where artificial intelligence technology is held to a high standard, as it should be. It’s also where the risk of generating deep fakes is evaluated.
Deep fakes are hyper-realistic artificial outputs that can be almost indistinguishable from genuine data, making it crucial to scrutinize the generated output carefully. The cycle of training and validation continues in an iterative loop until the model meets the expected criteria of proficiency, maintaining a balance between innovation and ethical considerations.
Also Read: Artificial Intelligence the self-designing machine
Types of Generative Models
Generative Adversarial Networks (GANs) have been a revolutionary advancement in generative AI technology. They consist of two neural networks: one for generating new data and another for discriminating or evaluating that data.
These networks work in opposition, in a sort of ongoing contest, to create more and more refined outputs. The use of neural network techniques in this dual architecture has been instrumental in many generative AI capabilities, from creating high-quality images to simulating complex systems.
Variational Autoencoders (VAEs) offer another approach to data generation. Unlike GANs, VAEs focus on creating data that is statistically similar to the input data. They are well-suited for applications where the generated data needs to closely match the training set, such as image manipulation tasks. VAEs use neural network techniques to understand the probabilistic distribution of the data, ensuring that the generated output aligns with the input.
Another significant category includes Transformer models, which are particularly adept at handling sequential data like text. These models, including popular GPT models, have shown extraordinary proficiency in natural language processing tasks.
Whether it’s translating languages, generating human-like text, or functioning as a speech model, the transformer architecture is designed to deal with the intricacies and complexities of language. By using multiple layers and attention mechanisms, these models excel in tasks that require a nuanced understanding of context, thus expanding the realm of what generative AI technology can accomplish.
Also Read: Introduction to Generative Adversarial Networks (GANs)
Generative AI in Art and Design
In the fields of art and design, generative AI has emerged as a groundbreaking asset for both exploration and creation. Designers now have access to special tools that employ generative design algorithms to quickly iterate through a multitude of design possibilities.
By setting specific parameters, such as material constraints or aesthetic considerations, these tools generate a range of options for the designer to choose from. This rapid iteration not only speeds up the design process but also results in creative content that might be beyond the reach of traditional methods.
Visual artists are also embracing generative AI to expand their creative horizons. Generative models, including some of the larger models specifically developed for image generation, offer artists an array of options to explore new compositions, color schemes, and even whole new styles.
These models act as a form of collaborative intelligence, working alongside the artist to discover and generate fresh, AI-generated content. This opens up a whole new realm of artistic exploration, allowing artists to venture into creative directions they might not have thought of on their own.
When it comes to music composition, generative AI is proving to be an invaluable creative assistant. Algorithms designed for music generation can create melodies, harmonies, or even entire compositions based on an initial musical idea provided by the composer.
This capacity for generating complex musical content is revolutionizing the way musicians work, speeding up the creative process considerably. Just like in visual arts and design, the use of generative AI in music allows for a broader scope of creative exploration, introducing a myriad of new opportunities for artists to craft their work.
Also Read: Redefining Art with Generative AI
The Impact of Generative AI on Writing and Journalism
In the fast-paced world of journalism and content creation, generative AI is steadily carving out a significant role for itself. One of its most impactful contributions is in automating the generation of certain types of reports. For example, financial summaries and weather forecasts, which usually follow a standardized format, can be quickly and accurately produced by generative AI.
This automation frees up human journalists to focus on more complex and nuanced work, such as investigative reporting or in-depth analysis. The integration of AI in this manner allows for a more efficient newsroom, where each type of task—routine or complex—is allocated to the most suitable agent, whether it’s a machine or a human.
Generative AI also offers a treasure trove of capabilities in the realm of creative writing. It can act as a sophisticated writing assistant, capable of generating plot ideas for narratives or coming up with elegant phrasings for non-fiction pieces.
With AI algorithms getting increasingly adept at understanding human language and mimicking creativity, writers find themselves with a powerful tool for augmenting their creative process. Imagine a writer suffering from writer’s block; a generative AI can propose a surprising plot twist or suggest a persuasive argument, serving as a catalyst for the human writer’s own creativity.
The integration of generative AI in journalism and writing doesn’t come without its set of challenges and responsibilities. Human oversight remains crucial. Editors and journalists must meticulously vet any AI-generated content for various factors like accuracy, ethical implications, and overall quality.
An algorithm, no matter how advanced, might lack the nuance to understand the complexities of human bias or the potential repercussions of inaccurate reporting. Therefore, before any AI-generated content is made public, it must go through a rigorous human-led editorial process to ensure that it meets the high standards expected in journalistic and creative works.
Generative AI Applications in Health Care
Generative AI is emerging as a powerful force in healthcare, significantly impacting various aspects including diagnostics, treatment planning, and drug discovery. When it comes to diagnostics, generative models are particularly skilled at converting raw imaging data into intricate medical images.
This is especially useful for healthcare organizations that require quick and precise diagnoses for effective patient treatment. The ability to generate these high-quality images from healthcare datasets not only facilitates early detection of diseases but also enhances healthcare coverage by making diagnostic services more efficient and widely accessible.
The pharmaceutical sector is another area that’s reaping the benefits of generative AI. By predicting the interactions between various chemical compounds, these models speed up the process of drug discovery. This is immensely valuable for healthcare organizations focused on research and development.
The use of generative AI minimizes the time and resources traditionally needed for discovering new medicines, allowing scientists to focus on validating the most promising candidates. This can lead to faster approvals and better healthcare patient service as effective drugs reach the market more quickly.
In the area of personalized medicine, generative AI has shown considerable promise in tailoring treatment plans to individual patients. By analyzing extensive healthcare datasets that include a patient’s medical history and current conditions, these algorithms can recommend personalized treatment regimens. This individualized approach is a boon for enhancing patient experiences, as it increases the likelihood of successful medical interventions.
Some advanced models even offer conversational search experiences, allowing healthcare providers to input patient data in a natural, conversational manner to receive tailored treatment options. The inclusion of generative AI in personalized medicine has the potential to revolutionize how healthcare is delivered, making it more effective and attuned to individual patient needs.
Real-world Use Cases
Generative AI has successfully transitioned from being purely experimental to having wide-reaching real-world applications. In the agriculture sector, the technology is increasingly relied upon for genome analysis.
Here, generative models analyze the genetic makeup of various crops to predict and engineer strains that are more resistant to pests and diseases. This kind of genetic modeling not only improves the resilience of crops but also has the potential to increase yield, a crucial consideration for global food security.
The field of architecture is another area benefiting from the capabilities of generative AI. Architects use generative algorithms to automatically produce a range of building layouts that meet specific constraints, such as spatial limitations or sustainability goals.
By using AI in the conceptual phase, architects can quickly explore a myriad of design options. This doesn’t just save time but also encourages innovative design solutions that might not have been possible—or even conceivable—using traditional design methods.
The entertainment industry is also tapping into the potential of generative AI. In video gaming, the technology is employed to create ultra-realistic avatars and gaming environments, adding an extra layer of immersion and complexity to gameplay. Outside of gaming, some forward-thinking companies are leveraging AI to create virtual influencers for marketing and brand promotion.
These AI-generated personalities can interact with audiences in real time, offer product recommendations, and even have their own social media presence, all designed to engage customers in a novel way. The applicability of generative AI in these various sectors showcases its versatility and its growing importance in shaping the modern world.
The Dark Side of Generative AI
While generative AI offers a range of benefits across different sectors, it also comes with its own set of risks. One of the most talked-about concerns is the emergence of deepfakes, which are highly convincing but fake audio and video clips. These can be potent tools for disinformation campaigns, identity theft, or political manipulation. The convincing nature of deepfakes poses a threat to societal trust and can even destabilize democratic systems.
Ethical concerns extend into the realm of intellectual property and plagiarism as well. Since generative models have the capability to create diverse types of creative content, it raises questions about the originality of such outputs. Is a piece of AI-generated music eligible for copyright? Who owns the rights to AI-generated art or articles? These questions pose significant challenges to existing legal frameworks around creativity and ownership.
Job displacement is also a significant concern as generative AI technologies advance. These technologies can perform tasks that were previously reserved for human experts, especially in creative and analytical fields. This leads to a growing apprehension about the role of human labor in these sectors. The risk extends to the creation and spread of misinformation too.
Generative AI can produce fake news articles or misleading reports that could appear highly credible. Misinformation of this kind can rapidly proliferate through social media platforms, causing public confusion and eroding trust in legitimate news sources. So, while generative AI’s capabilities are undoubtedly transformative, they also require thoughtful governance to manage the associated risks.
Ethical Considerations of Generative AI
Ethical questions around generative AI loom large, especially as these technologies become more prevalent in various sectors. One of the most pressing issues is accountability.
When a generative model churns out harmful or misleading content—be it fake news, manipulated images, or biased reports—the question arises: Who is responsible? Is it the team that trained the model, the organization that deployed it, or the users who interact with it? The answer isn’t straightforward but has far-reaching implications for legal systems that may need to adapt to a landscape where machines create content.
Bias in generative AI models is another ethical minefield. Often, these models are trained on large datasets sourced from the internet or other real-world scenarios. If these datasets contain biases—gender bias, racial bias, or any other form of societal discrimination—there’s a strong chance the model will perpetuate these biases in its generated content.
For example, a language model trained on a dataset that includes sexist language is more likely to produce sexist content. This not only perpetuates harmful stereotypes but also raises questions about the ethical considerations that go into selecting and curating training data.
The ethical challenges extend to issues of consent and data privacy, particularly when models are trained on user-generated content. For example, if a generative model is trained on photographs shared on social media platforms or articles posted on personal blogs, it raises questions about whether the people who created that content have in effect consented to their data being used in this manner.
This comes into sharper focus when the data in question might be sensitive or personal in nature. Navigating these ethical waters requires a well-thought-out approach that respects individual rights while also fostering innovation. It’s a delicate balance that requires ongoing dialogue among technologists, ethicists, lawmakers, and the public.
The Future of Generative AI
It’s clear that generative AI will play an increasingly integral role in our day-to-day experiences. Advances in algorithms and increased computational power will pave the way for models that can generate content with greater accuracy and creativity. As machine learning techniques become more sophisticated, we can expect to see generative AI applications that go beyond what we currently envision.
It’s not just about better chatbots or more convincing virtual realities; we could see breakthroughs in fields as diverse as medicine, urban planning, and environmental sustainability.
Yet, as these technologies evolve, the urgency for ethical oversight and responsible policies grows in tandem. The future might bring even more potent forms of generative AI that can impact society in profound ways—both positive and negative.
This amplifies the need for a framework that ensures these technologies are developed and deployed responsibly. It’s crucial to have guidelines that safeguard against harmful uses of the technology, from misinformation to ethical and privacy concerns. It’s not just about what the technology can do, but also about what it should and shouldn’t be allowed to do.
The landscape of generative AI is both exhilarating and fraught with challenges. The technology promises to revolutionize a range of industries, offering tools that can generate a wide array of content and solutions.
The same capabilities that make it so promising also make it a subject of ethical and practical scrutiny. Balancing the potential benefits with the inherent risks will require ongoing, thoughtful conversations among all stakeholders, including technologists, policymakers, and the broader public.